Accelerate next-gen Al training with realistic synthetic sensor data
- henrikbattke
- Mar 17
- 2 min read
Updated: Apr 13
In today’s AI-driven world, object recognition has become an essential component of various industries, from autonomous vehicles to industrial automation. The power of deep neural networks (DNN) has revolutionized image recognition, classification, and tracking. However, the foundation of reliable AI models lies in high-quality training data.
While synthetic training data cannot fully replace real-world data, it serves as a valuable tool for efficiently developing classification and tracking algorithms. It significantly reduces the cost, time, and personnel requirements involved in collecting and labeling large datasets.
One of the most significant challenges in AI training is the limited availability of sensor data or images for specific object classes. In many cases, obtaining extensive datasets is impractical or too expensive. This is where synthetic data comes into play. By leveraging advanced modeling, staging, and rendering techniques, AI developers can create highly realistic scenes that replicate real-world conditions and generate various types of sensor data.
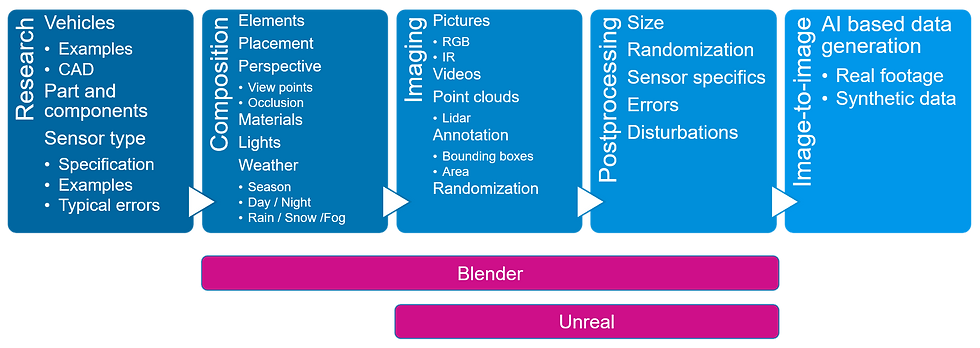
A Marder IVF in different variants of a lidar simulation. The emitter and sensor do not have to be in the same position. Shadowing can also be simulated realistically.
In the initial phases of AI development, teams often experiment with different sensor types and object recognition models to determine the most effective approach. Synthetic AI training data provides a rapid and scalable way to test and refine these models before committing to costly data collection processes. This accelerates project timelines and enhances model performance before real-world deployment.
A forest scene with a T72
As AI technology continues to advance, synthetic training data will play an increasingly vital role in bridging data gaps, improving model accuracy, and reducing development costs. By integrating synthetic sensor data into AI workflows, organizations can enhance the efficiency and effectiveness of their object recognition systems, ultimately driving innovation and technological progress.
By embracing the potential of synthetic data, businesses and researchers can develop next-generation AI solutions with greater speed, precision, and scalability.
Schwerer Waffenträger in different synthetic sensor views
Comments